Ivan Smirnov, Leading Research Fellow of the Laboratory of Computational Social Sciences at the Institute of Education of HSE University, has created a computer model that can distinguish high academic achievers from lower ones based on their social media posts. The prediction model uses a mathematical textual analysis that registers users’ vocabulary (its range and the semantic fields from which concepts are taken), characters and symbols, post length, and word length.
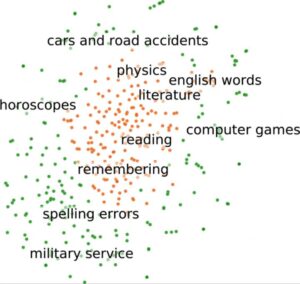
Thematic clusters: t-SNE representation of the words with the highest and lowest scores from the training data set
CREDIT
I.Smirnov
Every word has its own rating (a kind of IQ). Scientific and cultural topics, English words, and words and posts that are longer in length rank highly and serve as indicators of good academic performance. An abundance of emojis, words or whole phrases written in capital letters, and vocabulary related to horoscopes, driving, and military service indicate lower grades in school. At the same time, posts can be quite short–even tweets are quite informative. The study was supported by a grant from the Russian Science Foundation (RSF), and an article detailing the study’s results was published in EPJ Data Science.
Smirnov’s study used a representative sample of data from HSE University’s longitudinal cohort panel study, ‘Educational and Career Trajectories’ (TrEC). The study traces the career paths of 4,400 students in 42 Russian regions from high schools participating in PISA (the Programme for International Students Assessment). The study data also includes data about the students’ VK accounts (3,483 of the student participants consented to provide this information).
‘Since this kind of data, in combination with digital traces, is difficult to obtain, it is almost never used,’ Smirnov says. Meanwhile, this kind of dataset allows you to develop a reliable model that can be applied to other settings. And the results can be extrapolated to all other students high school students and middle school students.
Posts from publicly viewable VK pages were used as a training sample–this included a total of 130,575 posts from 2,468 subjects who took the PISA test in 2012. The test allowed the researcher to assess a student’s academic aptitude as well as their ability to apply their knowledge in practice. The study included only publicly visible VK posts from consenting participants.
When developing and testing the model from the PISA test, only students’ reading scores were used an indicator of academic aptitude, although there are three tests in total: reading, mathematics, and science. PISA defines reading literacy as ‘understanding, using, reflecting on and engaging with written texts in order to achieve one’s goals, to develop one’s knowledge and potential, and to participate in society.’ The exam has six proficiency levels. Students who score a 2 are considered to meet only the basic, minimum level, while those who score a 5 or 6 are considered to be strong students.
In the study, unsupervised machine learning with word vector representations was performed on VK post corpus (totaling 1.9 billion words, with 2.5 million unique words). It was combined with a simpler supervised machine learning model that was trained in individual positions and taught to predict PISA scores.
‘We represented each post as a 300-dimensional vector by averaging over vector representations of all its constituent words,’ Smirnov writes. ‘These post representations were used to train a linear regression model to predict the PISA scores of the posts’ authors.’
By ‘predict’, the researcher does not refer to future forecasting, but rather the correlation between the calculated results and the real scores students earned on the PISA exam, as well as their USE scores (which are publicly available online in aggregated form–i.e., average scores per school). In the preliminary phase, the model learned how to predict the PISA data. In the final model, the calculations were checked against the USE results of high school graduates and university entrants.
The final model was supposed to be able to reliably recognize whether a strong student or a weak student had written a particular social media post, or in other words, differentiate the subjects according to their academic performance. After the training period, the model was able to distinguish posts written by students who scored highly or poorly on PISA (levels 5-6 and levels 0-1) with an accuracy of 93.7%. As for the comparability of PISA and the USE, although these two tests differ, studies have shown that students’ scores for the two tests strongly correlate with each other.
‘The model was trained using PISA data, and we looked at the correlation between the predicted and the real PISA scores (which are available in the TrEC study),’ Smirnov explains. ‘With the USE things gets more complicated: since the model does not know anything about the unified exams, it predicted the PISA scores as before. But if we assume that the USE and PISA measure the same thing — academic performance — then the higher the predicted PISA results are, the higher the USE results should be.’ And the fact that the model learned to predict one thing and can predict another is quite interesting in itself, Smirnov notes.
However, this also needed to be verified, so the model was then applied to 914 Russian high schools (located in St. Petersburg, Samara and Tomsk; this set included almost 39,000 users who created 1.1 million posts) and one hundred of Russia’s largest universities (115,800 people; 6.5 million posts) to measure the academic performance of students at these institutions.
It turned out that ‘predicted academic performance is closely related to USE scores,’ says Smirnov. ‘The correlation coefficient is between 0.49 and 0.6. And in the case of universities, when the predicted academic performance and USE scores of applicants were compared (the information is available in HSE’s ongoing University Admissions Quality Monitoring study), then the results also demonstrated a strong connection. The correlation coefficient is 0.83, which is significantly higher than for high schools, because there is more data.’
But can the model be applied to other social media sites? ‘I checked what would happen if, instead of posts on VK, we gave the model tweets written by the same users,’ Smirnov says. ‘It turned out that the quality of the model does not significantly decrease.’ But since a sufficient number of twitter accounts were available only for the university dataset (2,836), the analysis was performed only on this set.
It is important that the model worked successfully on datasets of different social media sites, such as VK and Twitter, thereby proving that is can be effective in different contexts. This means that it can be applied widely. In addition, the model can be used to predict very different characteristics, from student academic performance to income or depression.
Smirnov’s study used a representative sample of data from HSE University’s longitudinal cohort panel study, ‘Educational and Career Trajectories’ (TrEC). The study traces the career paths of 4,400 students in 42 Russian regions from high schools participating in PISA (the Programme for International Students Assessment). The study data also includes data about the students’ VK accounts (3,483 of the student participants consented to provide this information).
‘Since this kind of data, in combination with digital traces, is difficult to obtain, it is almost never used,’ Smirnov says. Meanwhile, this kind of dataset allows you to develop a reliable model that can be applied to other settings. And the results can be extrapolated to all other students–high school students and middle school students.
Posts from publicly viewable VK pages were used as a training sample–this included a total of 130,575 posts from 2,468 subjects who took the PISA test in 2012. The test allowed the researcher to assess a student’s academic aptitude as well as their ability to apply their knowledge in practice. The study included only publicly visible VK posts from consenting participants.
It is important that the scores on the standardized PISA and USE tests were used as an academic aptitude metric. This gives a more objective picture than assessment mechanisms that are school-specific (such as grades).
When developing and testing the model from the PISA test, only students’ reading scores were used an indicator of academic aptitude, although there are three tests in total: reading, mathematics, and science. PISA defines reading literacy as ‘understanding, using, reflecting on and engaging with written texts in order to achieve one’s goals, to develop one’s knowledge and potential, and to participate in society.’ The exam has six proficiency levels. Students who score a 2 are considered to meet only the basic, minimum level, while those who score a 5 or 6 are considered to be strong students.
In the study, unsupervised machine learning with word vector representations was performed on VK post corpus (totaling 1.9 billion words, with 2.5 million unique words). It was combined with a simpler supervised machine learning model that was trained in individual positions and taught to predict PISA scores.
Word vector representations, or word embedding, is a numeric vector of a fixed size that describes some features of a word or their sequence. Embedding is often used for automated word processing. In Smirnov’s research, the fastText system was used since it is particularly conducive to working with Russian-language text.
‘We represented each post as a 300-dimensional vector by averaging over vector representations of all its constituent words,’ Smirnov writes. ‘These post representations were used to train a linear regression model to predict the PISA scores of the posts’ authors.’
By ‘predict’, the researcher does not refer to future forecasting, but rather the correlation between the calculated results and the real scores students earned on the PISA exam, as well as their USE scores (which are publicly available online in aggregated form–i.e., average scores per school). In the preliminary phase, the model learned how to predict the PISA data. In the final model, the calculations were checked against the USE results of high school graduates and university entrants.
Results
First, Smirnov highlighted the general textual features of posts in relation to the academic performance of their authors (Fig. 1). The use of capitalized words (-0.08), emojis (-0.06), and exclamations (-0.04) were found to be negatively correlated with academic performance. The use of the Latin characters, average post and word length, vocabulary size, and entropy of users’ texts on the other hand, were found to positively correlate with academic performance (from 0.07 to 0.16, respectively).
It was also confirmed that students with different levels of academic performance have different vocabulary ranges. Smirnov explored the resulting model by selecting 400 words with the highest and lowest scores that appear at least 5 times in the training corpus. Thematic clusters were identified and visualized (Fig. 2).
The clusters with the highest scores (in orange) include:
- English words (above, saying, yours, must);
- Words related to literature (Bradbury, Fahrenheit, Orwell, Huxley, Faulkner, Nabokov, Brodsky, Camus, Mann);
- Concepts related to reading (read, publish, book, volume);
- Terms and names related to physics (Universe, quantum, theory, Einstein, Newton, Hawking);
- Words related to thought processes (thinking, memorizing).
Clusters with low scores (in green) include misspelled words, names of popular computer games, concepts related to military service (army, oath, etc.), horoscope terms (Aries, Sagittarius), and words related to driving and car accidents (collision, traffic police, wheels, tuning).
Smirnov calculated the coefficients for all 2.5 million words of the vector model and made them available for further study. Interestingly, even words that are rarely found in a training dataset can predict academic performance. For example, even if the name ‘Newt’ (as in the Harry Potter character, Newt Scamander) never appears in the training dataset, the model might assign a higher rating to posts that contain it. This will happen if the model learns that words from novel series are used by high-achieving students, and, through unsupervised learning, ‘intuit’ that that the name ‘Newt’ belongs to this category (that is, the word is closely situated to other concepts from Harry Potter in the vector space).
Source: NATIONAL RESEARCH UNIVERSITY HIGHER SCHOOL OF ECONOMICS